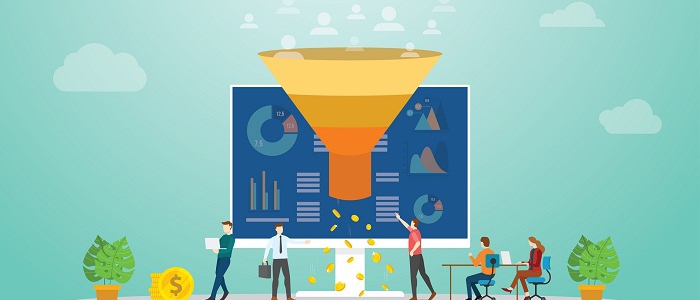
Lead conversion probability is finding the win likelihood of each incoming lead generated for your business. To do this manually, sales managers usually apply their gut instinct that they have gathered from their historical lead conversion experience on the current profile of every lead to predicting. Even though this assumption based prediction help to a level, it will be better to utilize conversion probability methods based on user profile and mountain of historical conversion data available at CRM.
Weighted Probability
Weighted probability is far better than relying on personal assumptions. In this method, each profile factor chosen for calculating prediction will be assigned a boundary for the low and high categorization based on the historical conversion data.
For example, considering Industry as a profile factor, any lead with manufacturing profile attribute will be predicted for higher probability if the historical data claims 60% of leads converted are from manufacturing vertical. Likewise combining multiple factors, each lead can be assigned a unique probability score to predict the win likelihood.
Machine Learning
Machine learning is building and applying a prediction model based on algorithms to estimate the conversion probability of each lead. Once you have identified most relevant profile factors e.g, industry, sales team size – for predictions refined with data cleansing & statistical filtering, a prediction model will be built by machine learning to relate the vector of input factors to the resulting conversion probability.
By applying this model, each incoming lead will be assigned a unique probability to convert. This prediction model will be learning from the historical data continuously to refine its predictions. Typical algorithms for machine learning include logistic regression and random forests.
Both of these methods can be employed to compare the quality of the predictions and taking a longer historical data (say 12 months) for analysis will help achieve quality predictions.